Robust healthcare systems require skilled labor, and there’s evidence we’re short on medical experts. The Association of American Medical Colleges predicts a shortage of up to 139,000 physicians in the U.S. by 2033. Globally, there’s a projected shortfall of up to 10 million nurses by 2030, warns the International Council of Nurses. The world needs to start training new healthcare professionals—and to invest in technology that can help limit the impact of scarce medical workers.
Much of this technology will use Artificial Intelligence (AI) to confront the complexities of human health. To be clear, AI is no replacement for the medical workforce. But it can support medical professionals in powerful ways; in the hands of doctors, nurses, and technicians, AI is improving patient care across the globe. Here’s what everyone—from healthcare providers to policy-makers to patients—should know about artificial intelligence in healthcare.
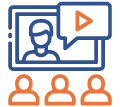
Ready to Transform Your Business with AI, NLP, and Automation?
Watch our on-demand webinar and discover how AI, NLP, and advanced cutting-edge automation technologies can supercharge your growth, streamline processes, and reduce costs.
Prominent Types Of Artificial Intelligence (AI) In Healthcare
Artificial intelligence is computer technology that simulates human actions. Humans are good at two things that computers usually aren’t: thinking and learning. With AI, machines are capable of learning from large stores of data, or even from human behavior. They can use that learning to make decisions—a form of “thinking.” These AI capabilities allow computers to solve real-life problems, rather than formal, rule-based problems—and one of the most common characteristics of real-life problems is that they are hard to solve.
As this differentiation between “real-life” and “formal” problems suggests, the key difference between AI computing and a more traditional model relates to the type of logic these systems use. Two types of logic concern us here:
- In Boolean logic, the output of a processing task is binary: It can be either yes or no, true or false, 1 or 0.
- Fuzzy logic is spectral (in the sense of a spectrum, not a ghost). Responses aren’t just yes or no. With fuzzy logic, there is a probability associated with the outcome, which is a value between 0 and 1 indicating the propensity of the truth of the outcome.
Fuzzy logic leads to the creation of new algorithms capable of far greater complexity than binary computing. In the healthcare field, a few key AI technologies are emerging as useful tools. Prominent artificial intelligence in healthcare includes:
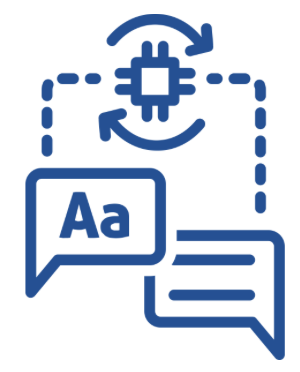
Natural Language Processing (NLP)
Natural language processing is an AI technology that allows machines to “understand” and interpret human language. That capability leads to many language-based capabilities. For example, some NLP systems scan news articles and then summarize them, creating instant article abstracts. Before the advent of AI, only humans could perform an interpretive and creative task like this. So, how do you build an NLP system? You start with a core AI technology: machine learning.
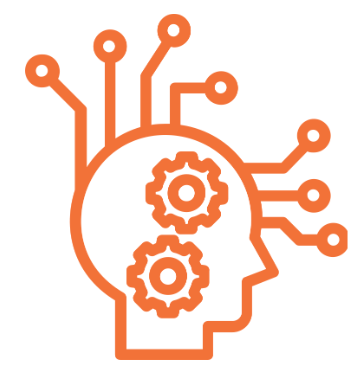
Machine Learning
Machine learning is just what it sounds like: A process by which computer models train on datasets to develop a form of knowledge or expertise. Machine learning has three parts: First, it trains through exposure to datasets. Then, it identifies patterns in that data. Using the knowledge obtained from these first two steps, AI with machine learning can make decisions—and help doctors make decisions. But to improve the AI tools that lead to greater health outcomes, computer scientists may employ a more powerful form of machine learning: deep learning.
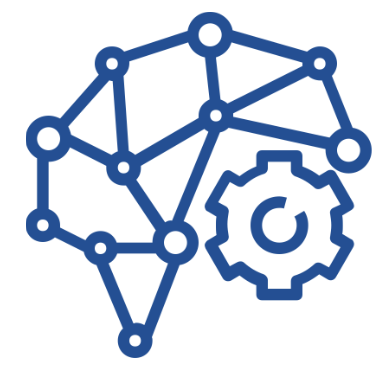
Deep Learning
Deep learning is a form of machine learning conducted on a particular architecture called a Deep Neural Network (DNN). These systems mimic the architecture of the human brain. When you learn a skill, you create an efficient electrochemical pathway between neurons—that’s what learning is. When you train a deep neural network, it creates efficient processing pathways between its artificial neurons.
To be clear, machine learning is a subset of artificial intelligence, and deep learning is a subset of machine learning. It’s just one way to achieve machine learning—but the neural connectivity model is capable of processing extremely large datasets and solving complex problems. In a high-risk industry like healthcare, you want the most accurate computational models available. That requires tremendous datasets. Compared to other forms of machine learning, deep learning achieves higher accuracy through its ability to train on larger datasets—that makes it an ideal technology for artificial intelligence in healthcare.
Compared to other forms of machine learning, deep learning achieves higher accuracy through its ability to train on larger datasets—that makes it an ideal technology for artificial intelligence in healthcare.
Benefits And Use Cases Of AI In Healthcare
Because AI can solve complex problems—and the human body is a complex system—this technology is improving medicine in a wide variety of applications. Here’s how AI helps healthcare providers in just a few examples.
1. AI In Medical Imaging And Diagnostics
Perhaps the most exciting benefits AI can bring to healthcare are in the realm of advanced diagnostics. At the forefront of these developments is the use of deep learning for cancer detection.
Deep learning leads to digital diagnostic tools that can identify subtle health indicators humans might miss, particularly in the field of medical imaging—and particularly early in the course of the disease, when visual indicators are harder to spot. You can train an AI model with a database of medical images, from CT scans to X-rays to photos of skin conditions. With this training, the system will identify patterns that are difficult or impossible to see with the naked eye. When confronted with a new medical image, this AI tool recognizes those patterns and deviations from those patterns. That allows it to flag abnormal biological markers for the human healthcare team, including a percentage probability of its own accuracy—what’s called a confidence score.
This confidence score helps with medical workflow. Practitioners set their own benchmarks for diagnostic confidence scores. They can decide that, if the tool diagnoses a tumor with a 95% probability, for instance, it’s time to proceed to treatment. But if the confidence score is only 60%, doctors may instead choose further testing as the next step.
2. Patient-Care AI Chatbots
Treatment doesn’t end when a patient walks out of the hospital or consultation room. Many conditions require ongoing treatment, and few healthcare providers have the staff to ensure that patients take their medications at the right time or continue their physical therapy exercises. AI chatbots can step in to help.
These app- or web-based conversational assistants use NLP to understand patient queries, either through text or, in phone-based systems, spoken language. They can send reminders when it’s time for another dose or answer frequently asked questions with personalized detail. When a patient asks a question the chatbot can’t handle, it can recognize the need for human assistance and pass the issue on to a practitioner.
3. Expanding Access To Care With AI Data Analytics
In many areas of the world, there simply aren’t enough doctors to go around. Lack of medical experts leads to worse health outcomes; in the United States, people who live in rural areas—where access to healthcare is more limited than in cities—face greater risk of death from heart disease, cancer, chronic lower respiratory disease, stroke, and unintentional injury than their urban counterparts.
Certainly, AI chatbots can help to fill the gap. Remote treatment, through videoconferencing, is another tech solution (albeit one that’s less dependent on AI). But the data analysis capabilities of AI can also identify areas that lack healthcare facilities. Governments and healthcare companies can use AI analysis models to determine locations where a new facility will serve the greatest number of people.
4. AI-Assisted Surgery
Surgical robots use AI to perform microsurgical procedures, creating tiny incisions with greater accuracy than the human hand can achieve. This application of AI is not just software; it also involves robotics. While this technology remains new, it’s already been proven in the field. In 2017, surgeons at the Dutch hospital Maastricht University Medical Center completed an advanced medical procedure with the help of an AI surgical robot. The system sutured blood vessels too small for human movements, down to just .03 millimeters in diameter.
5. Reading Medical Documents With IDP
Medical providers access records for each and every patient, and those files are often quite large, packed with key details that may contain clues for quick, accurate diagnoses. Given the volume of data in a medical file, certain details are easy to miss. One solution is Intelligent Document Processing (IDP), in which AI technologies like Computer Vision (CV), Optical Character Recognition (OCR), NLP, and machine learning categorize and extract key data from documents of all types.
At Nividous, we’ve trained machine learning models to identify medical documents—from historical data to medical tests to treatment reports—and to extract key information. These IDP systems provide doctors with the data they need to ask the right questions in their patient interviews. The same IDP systems can speed up record searches by identifying and categorizing every document in a patient’s file. And IDP is just one example of how hyperautomation on the Nividous platform uses AI to assist healthcare providers.
6. Predictive Care From AI Analysis
Every person is unique. One person may have grown up around smokers, another may suffer from metabolic syndrome, and a third may have a clean health background but occasionally experience strange symptoms for an unidentified reason. These three individuals may all come into the hospital for a sensation of chest pressure, but have entirely separate diagnoses and treatment plans.
The trouble is, unless a physician sits by the bedside and takes a detailed health history, the dramatic difference between these patients would not be apparent upon a standard examination. AI can help by analyzing patients’ histories and symptoms and using advanced algorithms to suggest possibilities that may elude doctors looking for the most common illnesses.
7. Customized Treatment Suggestions From Machine Learning
AI can also use extensive personal data to evaluate the likelihood that a patient will respond to a particular treatment, identify the side effects that treatment may cause the individual, and even calculate the potential risks and benefits of alternative treatments.
8. NLP-Assisted Transcription Of Patient Interactions
Sometimes, a patient may divulge something they hadn’t mentioned during earlier interactions, and other times they might dismissively mention something that could break the diagnostic case wide open. When every detail might make the difference between successful or unsuccessful treatment, hospitals can’t risk missing crucial information during conversations with the patient. With AI-assisted recordings and transcriptions, the system can not only take intelligent, formatted notes on every patient interaction, it can also flag relevant information for further review by doctors.
9. Physical And Mental Health Alerts Within AI Chatbots
Similarly, patients may mention important details while conversing with a chatbot — while setting up appointments, following up after procedures, or asking health questions, for example. If a patient seems “off” or like they may be struggling with something, the AI system can recognize these red flags and bring them to the attention of healthcare professionals who can help.
10. Clinical Decision Support With Expert System Analysis
Rudimentary rule-based systems — essentially, glorified flowcharts — have been used in diagnostics since the 1970s. While these rules can help physicians recognize familiar patterns that could lead to a diagnosis, they’re hardly foolproof, and they can’t even approach the accuracy of human doctors. And though image-based analysis can now outperform humans for cancer diagnosis, non-image-based diagnostics through AI is still an emerging technology.
For now, AI-assisted diagnostics — known as medical expert systems — support physicians’ decisions with rule-based and algorithmic suggestions for possible diagnoses. With further advancement, we may see a point where physicians begin to rely on expert system diagnoses more and more.
11. Updating Patient Records With RPA
The average nurse spends 25% of their time handling administrative tasks such as making changes to patient charts or ensuring all patient information is current and up-to-date before administering care.
While allowing automated bots to handle these tasks sounds less revolutionary than AI diagnostics or robotic surgery, the truth is that freeing up healthcare workers’ time could have powerful benefits for hospitals. Not only would workers have more time to work on human-centric projects, but offloading the tedious work to AI-assisted bots would likely reduce fatigue and lead to better patient care and fewer preventable errors.
12. Using RPA To Handle Routine Billing And Prior Authorizations
Billing and insurance are two more areas ripe for improvement with AI and automation. Many of the billing and insurance claims processes, including prior authorizations, are structurally similar and repetitive, and could easily be handled by AI-assisted bots.
13. Connected Hospitals For More Comprehensive Care
Today, a typical hospital operates as a giant unit that treats numerous conditions under one roof. If a patient switches to another hospital, they’ll be treated by entirely separate doctors, subjected to different diagnostic guidelines and treatment protocols, and have their records transferred to a different system.
The plan to solve this disconnection revolves around the idea of centralized hubs of care that treat only the most acute conditions, and smaller outposts that can handle specialized situations. All locations would be connected to the central, digital system so patient records can be transferred seamlessly and treatment remains consistent no matter where the patient might be located.
14. Identifying And Correcting Inaccuracies
Errors in medical codes, chart information, and health insurance claims could be matters of life and death. Even if patient care isn’t directly affected by the errors, the hospital could still be liable for any mistakes that slip through the cracks.
For the sake of patient health and hospital liability, it’s imperative to root out and solve data inaccuracies before they lead to other problems down the line. But poring over patient charts and claims manually soaks up a great deal of time and effort, and there is no guarantee a fatigued human worker would catch every mistake before approving the document. Using AI, the hospital could find and correct these errors with far greater speed and accuracy, reducing human involvement down to a simple, one-step approval process.
15. Less Burden On Patients And Staff
Ultimately, a combination of AI solutions should reduce the stress experienced by both patients and staff. Patients will have shorter wait times and more personalized care, while healthcare staff will benefit from fewer tedious administrative tasks and a more streamlined workflow.
A Closer Look At AI Diagnostics And Patient Experiences
The diagnostic capabilities of AI certainly hold plenty of promise. Hospitals are eager to reduce the burden on physicians, and AI companies are happy to assist by coming up with new and innovative tools.
Plus, if anything conjures the image of futuristic hospitals diagnosing and curing patients with the efficiency of science-fiction movies, it’s the field of AI diagnostics. This might not seem relevant from the perspective of a hospital or an AI developer, but it can actually have a significant effect on how patients perceive the medical care they receive. If advanced technology interests patients and improves their confidence in the hospital’s ability to provide quality care, that can translate to better patient outcomes for a number of reasons.
Patients will be more likely to come in for minor problems they may otherwise have ignored or tried to treat at home, for example, and they may also maintain more positive attitudes throughout the diagnosis and treatment processes. If AI tools make follow-ups more convenient, pleasant, or even exciting, patients will be more likely to adhere to post-treatment recommendations as well.
While patient attitudes toward AI-assisted diagnostics are not always positive, healthcare providers can encourage patients to think of AI tools as supplements to human care, rather than as replacements. With the right balance of capable AI technologies and person-to-person contact with physicians, healthcare providers can put patients’ minds at ease and even get them interested in improving their own health and wellness.
Here are just a few examples of the many AI diagnostics advances on the horizon that promise to make the often worrying, painful, and confusing process of receiving a diagnosis more pleasant for patients.
Convenient and painless skin lesion scans
We’ve mentioned how deep learning models can look at patient scans and detect anomalies before the human eye can perceive that something is wrong. Interestingly, this technology may soon be available inside smaller clinics and eventually patient homes, at least for external skin conditions. One area of interest is in handheld scanners that detect carcinomas and other forms of external cancer when passed over the skin.
Devices such as these can help dermatologists diagnose skin cancer earlier than they might have otherwise. In increasing the convenience and speed of scans, they also improve the likelihood that patients will scan for lesions more frequently, which also boosts the rate of early cancer detection. If painless scans can eventually replace — or reduce the rate of — painful biopsies, this would also improve the patient experience.
Faster and more comfortable X-rays
Though receiving X-rays isn’t painful by itself, many patients must wait on the table in uncomfortable positions, often while dealing with pain from fractures or other injuries. AI-driven X-ray machines are being developed to scan and process images more quickly and efficiently, reducing the scan time and lessening the need for repeat scans due to blurry images or suboptimal positioning. This translates to less time spent waiting on a cold, uncomfortable table for patients who are already worried and in pain.
At-home diagnosis and appointment check-ins with no wait times
Many people experiencing moderate injuries or illnesses — too severe to treat at home, but not severe enough to warrant a trip to the emergency room — delay making a doctor’s appointment or going to an urgent care clinic because the idea of waiting for hours in an overcrowded lobby with other contagious individuals doesn’t sound appealing. For these patients, at-home guidance from AI chatbots could make a huge difference.
Instead of delaying or avoiding care, patients could log on from home and exchange messages with an AI chatbot that can narrow down possible diagnoses, offer suitable options for treatment, and assist with appointment scheduling and check-ins to cut down on wait times.
The Future Of Automation In Healthcare
We believe AI will have far-reaching benefits in healthcare applications in the near future. Where the above technologies have not already been implemented, we expect hospitals to adopt new AI solutions within the next decade.
In cases where hospitals already have rudimentary AI solutions in place, advancements in AI will allow for even better efficiency and accuracy for patient care and administrative duties. For example, many hospitals already use speech capture and text recognition to transcribe patient interactions and record clinical notes, and we expect the use of these solutions to increase.
Using machine learning, AI will be the primary technology behind the advancement of precision medicine, which would dramatically benefit patient care. Though using AI for diagnostics and the augmentation of treatment recommendations has had its share of challenges, we anticipate AI will eventually pull ahead in those areas. Due to promising advancements in AI for image analysis, we believe we’ll soon see widespread usage of machines for reading radiology and pathology images.
Many people believe the greatest challenge in building AI solutions for healthcare applications is making the technology capable enough to be useful, but this isn’t necessarily the case. In many regards, we have passed this “proof of concept” stage already. Rather, the steepest hill to climb will be ensuring hospitals can adopt AI solutions for their everyday clinical operations.
We have passed the “proof of concept” stage already; now the steepest hill to climb will be ensuring hospitals can adopt AI solutions for their everyday clinical operations.
Before a hospital can adopt a custom solution, the AI program must achieve approval from regulators, integrate seamlessly with existing hospital systems, operate in parallel with AI systems from other hospitals, and be intuitive and easy for physicians to use. We expect advancements in AI will outpace adoption because of these issues, at least for the next few years. Inside of a decade, however, hospitals will likely become accustomed to passing these hurdles, and more widespread AI adoption can occur.
AI And Healthcare On The Nividous Platform
Artificial intelligence combined with Robotic Process Automation (RPA) and Business Process Management (BPM) leads to intelligent automation, in which a digital tool like the Nividous platform automates both individual tasks and complete, end-to-end processes, orchestrating work between RPA bots and human staff. Here are a few examples of Nividous intelligent automation in the healthcare industry:
- Nividous created patient-facing chatbots and voicebots to automate scheduling, improving process efficiency by 60%. Read the case study.
- A leading specialty healthcare company used the Nividous platform to automate data extraction and review for patient records. It further automated its claim submission processes. With intelligent automation, this provider achieved an 80% reduction in manual patient data processing—and cut operational costs by 45%. Read the case study.
- Medical coding can be costly and time-consuming. A major wound care company used Nividous’ smart RPA bots to automate its coding and billing processes, leading to a 90% improvement in accuracy, a 95% reduction in manual work, and turnaround-time improvement of 85%. Read the case study.
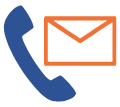
Artificial intelligence in healthcare is transformative, as these examples demonstrate. Ready to try it at your medical office?
Contact Nividous to discuss your unique process challenges—and find AI-driven solutions.